Book Downloads Hub Reads Ebooks Online eBook Librarys Digital Books Store Download Book Pdfs Bookworm Downloads Free Books Downloads Epub Book Collection Pdf Book Vault Read and Download Books Open Source Book Library Best Book Downloads Brian Godawa Yacub Saafir Aj Skelly Jodi Tyler Katy Shaw Peter Schreiner Walter C Parker Tappei Nagatsuki
Do you want to contribute by writing guest posts on this blog?
Please contact us and send us a resume of previous articles that you have written.
Unraveling the Mystery: Nonlinear Dimensionality Reduction Techniques

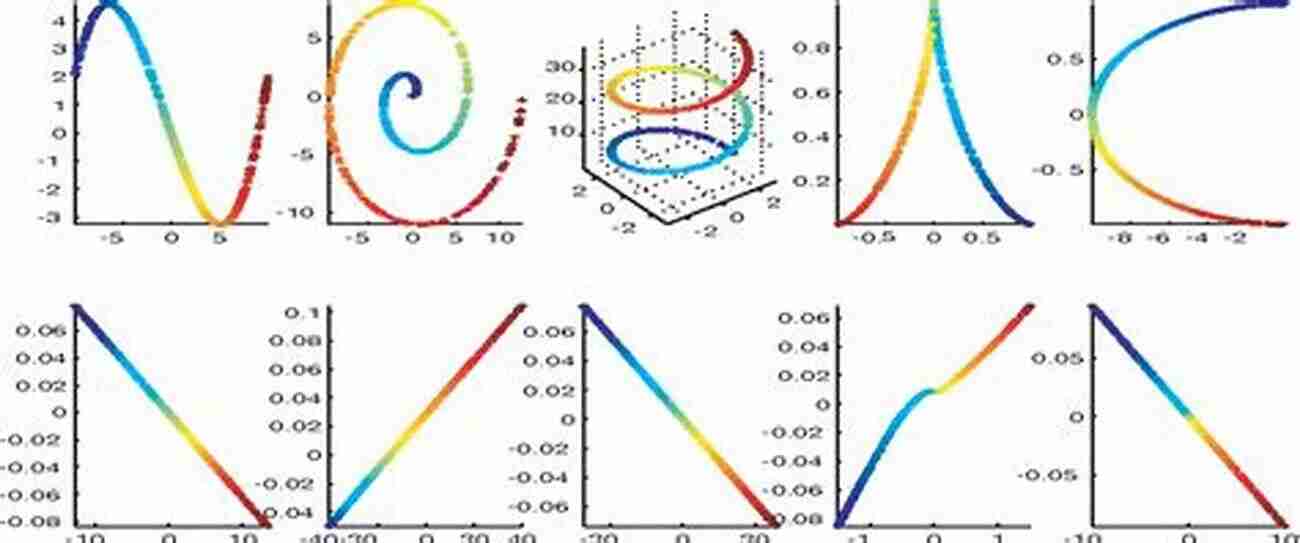
Are you tired of dealing with high-dimensional data that's difficult to visualize or comprehend? Nonlinear dimensionality reduction techniques might just be the solution you've been searching for. In this article, we will explore the intricacies and benefits of these techniques, guiding you through the world of data analysis and visualization.
The Importance of Dimensionality Reduction
Dimensionality reduction is an essential task in data analysis as it aims to transform high-dimensional data into a lower-dimensional representation while preserving its meaningful structure. By reducing the dimensionality of the data, we can simplify its complexity and gain valuable insights that were previously hidden in the overwhelming number of features or variables.
Traditional dimensionality reduction techniques, such as Principal Component Analysis (PCA),assume a linear relationship among the variables. However, many real-world datasets exhibit nonlinear relationships, making linear techniques less effective. This is where nonlinear dimensionality reduction techniques come into play, offering a more flexible approach to capture complex data patterns.
4.5 out of 5
Language | : | English |
File size | : | 13662 KB |
Screen Reader | : | Supported |
Print length | : | 263 pages |
Understanding Nonlinear Dimensionality Reduction Techniques
Nonlinear dimensionality reduction techniques are designed to address the limitations of linear methods by considering complex data dependencies. These techniques aim to find a lower-dimensional representation of the data that preserves the key structure and relationships present within it.
Some popular nonlinear dimensionality reduction techniques include:
- Kernel Principal Component Analysis (KPCA): KPCA uses the kernel trick to implicitly map the data into a higher-dimensional feature space, where linear techniques, such as PCA, can be applied. By capturing the nonlinear structure in the original space, KPCA allows for better representation and analysis of the data.
- Locally Linear Embedding (LLE): LLE constructs a low-dimensional representation of the data by preserving local relationships. It works by defining a set of weights that linearly reconstruct each data point from its neighbors. LLE excels at capturing intrinsic geometric structure and nonlinear manifolds in the data.
- Isomap: Isomap aims to preserve the geodesic distances between data points. It constructs a graph-based representation of the data, where each point is connected to its nearest neighbors. By computing the shortest paths on this graph, Isomap retains the underlying manifold structure even for high-dimensional data.
The Advantages of Nonlinear Dimensionality Reduction Techniques
Nonlinear dimensionality reduction techniques offer several advantages over their linear counterparts. By capturing nonlinear relationships in the data, these techniques can better reveal complex patterns and structures that exist within high-dimensional datasets. This, in turn, can lead to more accurate classification, clustering, and visualization results.
Moreover, nonlinear techniques often perform better on real-world datasets, as they can handle irregularities and nonlinearities that are common in many domains, such as bioinformatics, computer vision, or social network analysis. They provide a more robust framework for analyzing and interpreting such data, paving the way for further in-depth investigations and discoveries.
Applications and Future Directions
Nonlinear dimensionality reduction techniques have been successfully applied in various fields, including image and speech recognition, anomaly detection, and gene expression analysis, to name just a few. Their versatility and ability to reveal hidden structures make them invaluable tools in many data-driven domains.
As the volume and complexity of data continue to grow, the need for effective nonlinear dimensionality reduction techniques becomes even more apparent. Researchers are constantly exploring new algorithms and methodologies to further enhance the performance and capabilities of these techniques. This ongoing research is promising and will undoubtedly open up new possibilities for analyzing and understanding complex datasets.
Nonlinear dimensionality reduction techniques represent a powerful approach to deal with high-dimensional data and extract meaningful insights. By going beyond the linear constraints of traditional methods, these techniques enable researchers and data scientists to uncover hidden structures and relationships in complex datasets.
As the field continues to evolve, it is crucial to stay updated with the latest advancements in nonlinear dimensionality reduction techniques, ensuring that we are equipped with the best tools to tackle the challenging problems posed by modern data analysis.
4.5 out of 5
Language | : | English |
File size | : | 13662 KB |
Screen Reader | : | Supported |
Print length | : | 263 pages |
This book proposes tools for analysis of multidimensional and metric data, by establishing a state-of-the-art of the existing solutions and developing new ones. It mainly focuses on visual exploration of these data by a human analyst, relying on a 2D or 3D scatter plot display obtained through Dimensionality Reduction.
Performing diagnosis of an energy system requires identifying relations between observed monitoring variables and the associated internal state of the system. Dimensionality reduction, which allows to represent visually a multidimensional dataset, constitutes a promising tool to help domain experts to analyse these relations. This book reviews existing techniques for visual data exploration and dimensionality reduction such as tSNE and Isomap, and proposes new solutions to challenges in that field.
In particular, it presents the new unsupervised technique ASKI and the supervised methods ClassNeRV and ClassJSE. Moreover, MING, a new approach for local map quality evaluation is also introduced. These methods are then applied to the representation of expert-designed fault indicators for smart-buildings, I-V curves for photovoltaic systems and acoustic signals for Li-ion batteries.
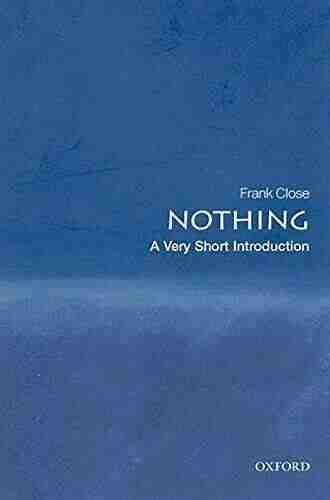

The Most Insightful and Liberating Experiences Found in...
When it comes to expanding our...
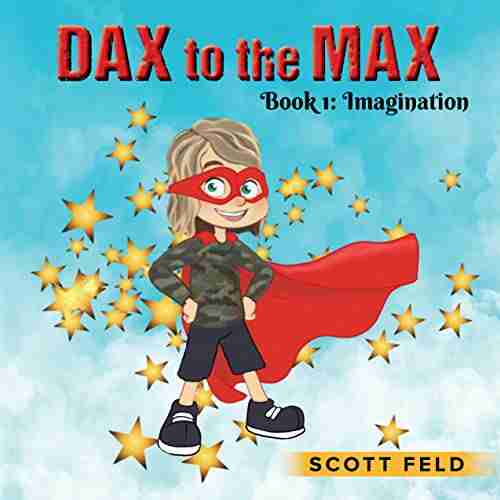

Dax To The Max Imagination: Unlock the Power of...
Welcome to the world of Dax To...
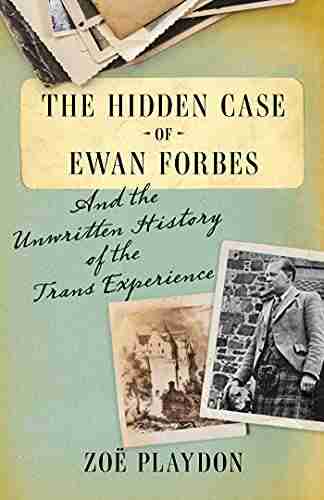

The Hidden Case of Ewan Forbes: Uncovering the Mystery...
Ewan Forbes: a...
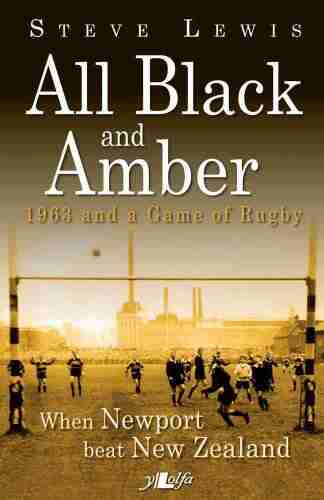

When Newport Beat New Zealand: A Historic Rugby Upset
The rivalry between Newport and New Zealand...
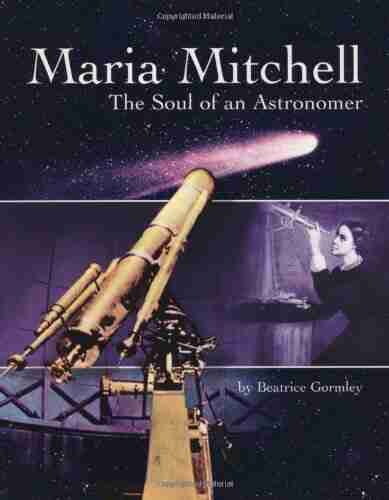

The Soul of an Astronomer: Women of Spirit
Astronomy, the study of...
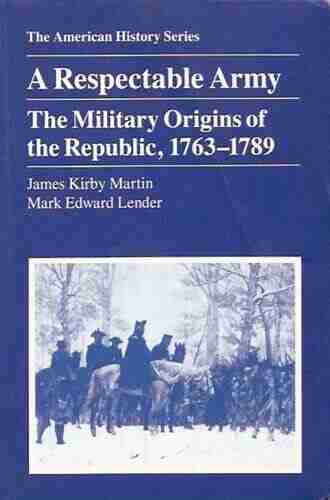

The Military Origins Of The Republic 1763-1789
When we think about the birth of the...
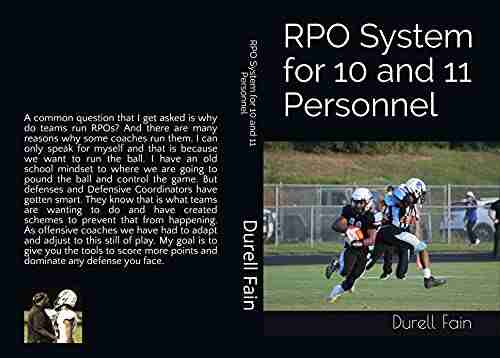

RPO System for 10 and 11 Personnel: Durell Fain
When it comes to...
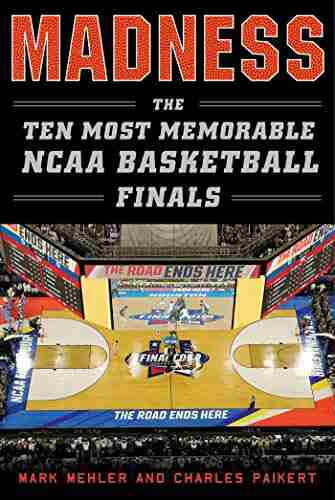

Madness: The Ten Most Memorable NCAA Basketball Finals
College basketball fans eagerly await the...
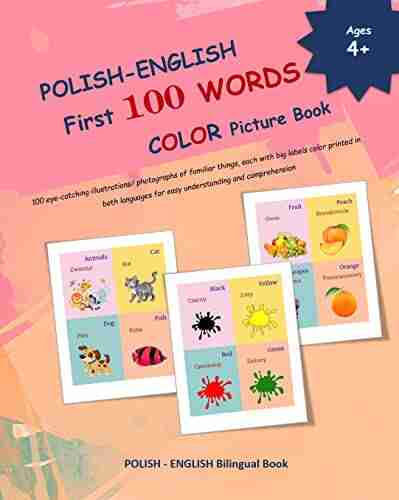

Discover the Magic of Polish: English First 100 Words,...
Are you ready to embark on a linguistic...
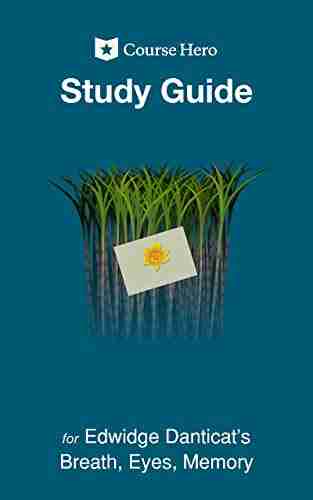

Unlock the Secrets of Edwidge Danticat's Breath, Eyes,...
Are you delving into the world...
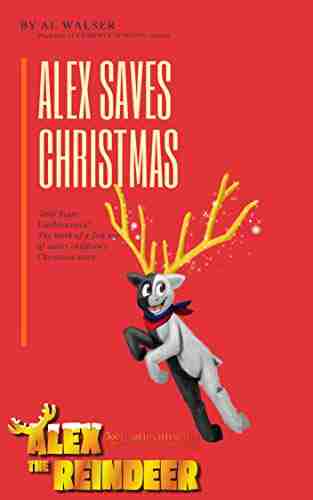

300 Years Liechtenstein: The Birth of Fish Out of Water...
Once upon a time, in the...
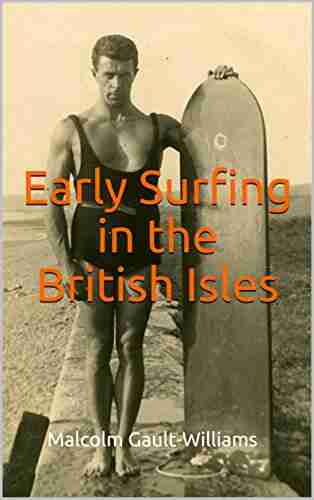

Exploring the Legendary Surfers of Early Surfing in the...
Surfing, a sport...
Light bulbAdvertise smarter! Our strategic ad space ensures maximum exposure. Reserve your spot today!
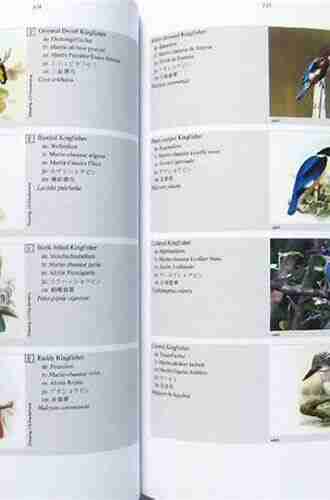

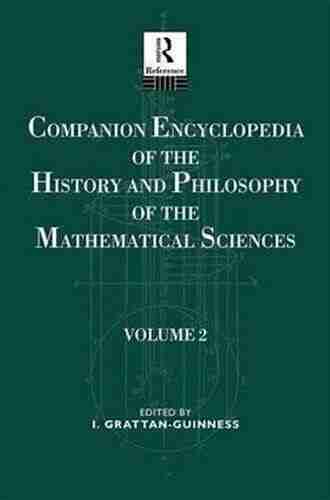

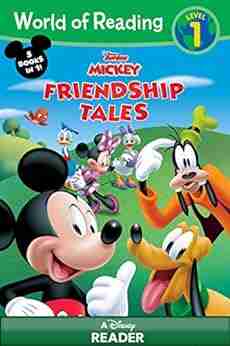

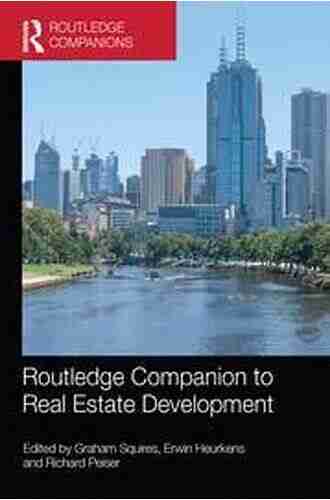

- Ernest ClineFollow ·14.1k
- Chadwick PowellFollow ·4k
- Gary CoxFollow ·16.7k
- Neil ParkerFollow ·19.4k
- Caleb LongFollow ·6.3k
- Logan CoxFollow ·10.9k
- Pete BlairFollow ·17.6k
- Jerry HayesFollow ·3.7k