Book Downloads Hub Reads Ebooks Online eBook Librarys Digital Books Store Download Book Pdfs Bookworm Downloads Free Books Downloads Epub Book Collection Pdf Book Vault Read and Download Books Open Source Book Library Best Book Downloads Subhadra Sen Gupta Dave Cousins Carina Gardner Mark Spencer Jeanne Wald Todd Keene Timberlake Usain Bolt Hadelin De Ponteves
Do you want to contribute by writing guest posts on this blog?
Please contact us and send us a resume of previous articles that you have written.
An Empirical Approach To Dimensionality Reduction And The Study Of Patterns

Are you fascinated by the possibilities of uncovering hidden patterns in complex data sets? Do you find yourself struggling with high-dimensional data that has too many variables to handle effectively? In this article, we will explore the concept of dimensionality reduction and its role in enabling the study of patterns in data through an empirical approach.
Understanding Dimensionality Reduction
Dimensionality reduction is a crucial technique used in data analysis and machine learning to reduce the number of variables or features in a dataset. It aims to simplify the data representation while preserving its important characteristics. By reducing the dimensionality, we can transform the data into a more manageable format without losing significant amounts of information.
High-dimensional data poses various challenges, such as increased computational requirements, overfitting, and difficulty in visualizing and interpreting the data. Dimensionality reduction methods tackle these challenges by identifying and eliminating irrelevant or redundant variables, thereby representing the data using a smaller number of informative features.
5 out of 5
Language | : | English |
File size | : | 7074 KB |
Text-to-Speech | : | Enabled |
Print length | : | 325 pages |
Lending | : | Enabled |
The Importance of Pattern Study
Patterns are inherent in many scientific fields, ranging from social sciences to biology, finance, and more. Recognizing and understanding patterns in data can uncover valuable insights, facilitate decision-making, and improve various processes.
However, patterns may not always be apparent due to the overwhelming complexity and noise present in high-dimensional datasets. Dimensionality reduction allows us to extract underlying patterns by reducing data complexity, improving the quality of analysis, and enhancing interpretability.
Empirical Approach to Dimensionality Reduction
An empirical approach to dimensionality reduction involves utilizing real-world data and practical techniques to reduce the dimensionality of a dataset. It focuses on the application of dimensionality reduction methods to extract meaningful patterns, rather than relying solely on theoretical assumptions.
One such popular empirical approach to dimensionality reduction is Principal Component Analysis (PCA). PCA aims to find linear combinations of the original variables that capture as much of the data's variation as possible. By projecting the data points onto the principal components, PCA reduces the number of dimensions while retaining the most informative features.
Benefits of Using an Empirical Approach
Employing an empirical approach to dimensionality reduction offers several advantages:
Simplifies Data Analysis:
By reducing the dimensionality of the data, an empirical approach simplifies data analysis tasks, making it easier to explore and interpret the patterns present in the dataset.
Improves Computational Efficiency:
High-dimensional data requires significant computational resources. Dimensionality reduction techniques reduce the number of variables, resulting in improved computational efficiency without compromising the quality of analysis.
Enhances Visualization:
Reducing the dimensionality of a dataset makes it easier to visualize the patterns. By transforming the data into a lower-dimensional space, we can plot and analyze the data in a more comprehensible manner.
An empirical approach to dimensionality reduction empowers researchers and data analysts to declutter complex data sets and uncover hidden patterns through practical techniques. By effectively reducing dimensionality, this approach simplifies analysis, improves computational efficiency, and enhances visualization. Understanding patterns is crucial for decision-making and insights in various scientific domains. So, consider applying an empirical approach to dimensionality reduction in your data analysis endeavors and embark on a journey of revealing intricate patterns.
5 out of 5
Language | : | English |
File size | : | 7074 KB |
Text-to-Speech | : | Enabled |
Print length | : | 325 pages |
Lending | : | Enabled |
This book addresses the most efficient methods of pattern analysis using wavelet decomposition. Readers will learn to analyze data in order to emphasize the differences between closely related patterns and then categorize them in a way that is useful to system users.
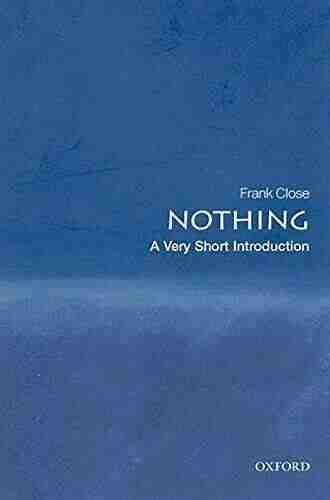

The Most Insightful and Liberating Experiences Found in...
When it comes to expanding our...
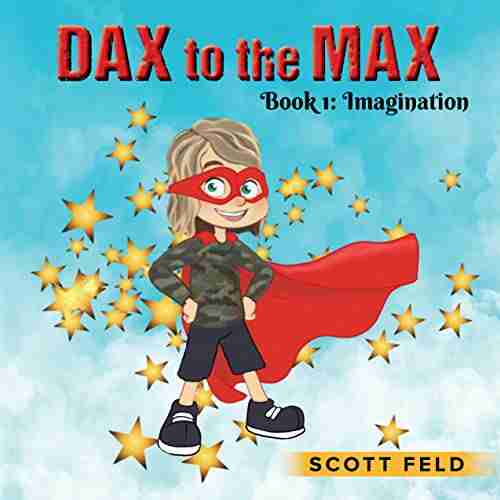

Dax To The Max Imagination: Unlock the Power of...
Welcome to the world of Dax To...
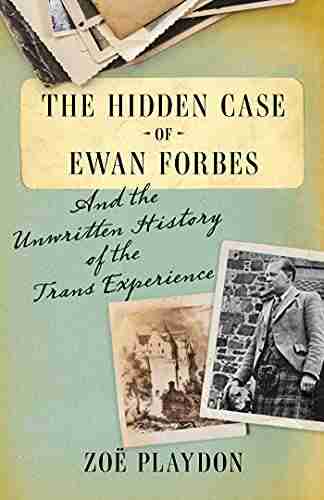

The Hidden Case of Ewan Forbes: Uncovering the Mystery...
Ewan Forbes: a...
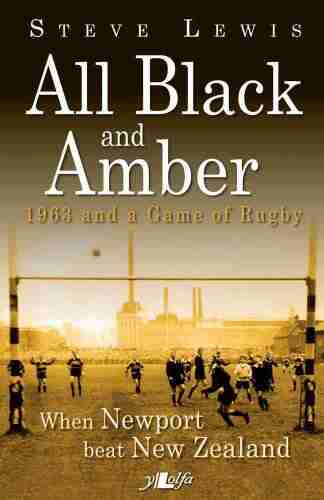

When Newport Beat New Zealand: A Historic Rugby Upset
The rivalry between Newport and New Zealand...
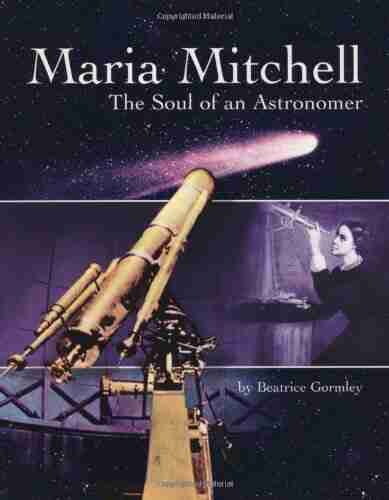

The Soul of an Astronomer: Women of Spirit
Astronomy, the study of...
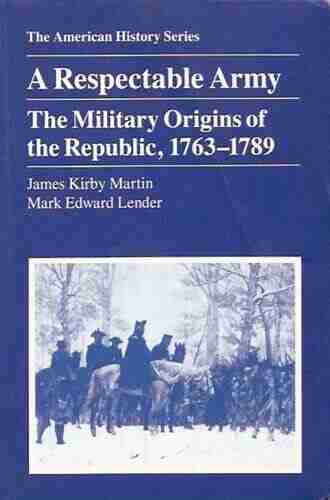

The Military Origins Of The Republic 1763-1789
When we think about the birth of the...
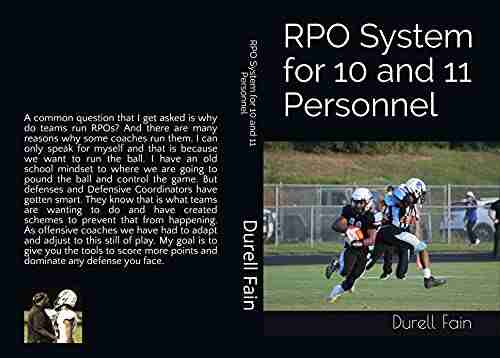

RPO System for 10 and 11 Personnel: Durell Fain
When it comes to...
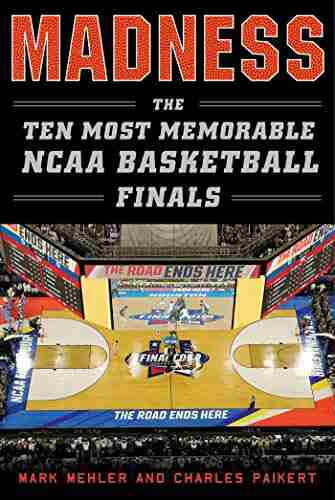

Madness: The Ten Most Memorable NCAA Basketball Finals
College basketball fans eagerly await the...
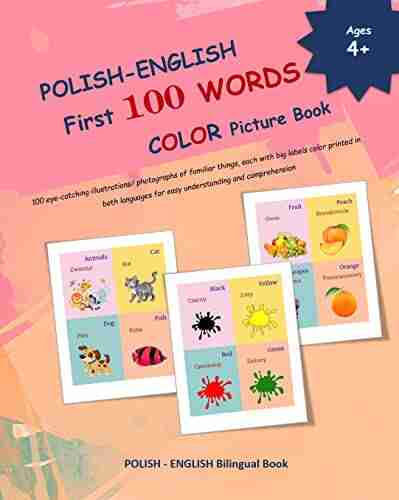

Discover the Magic of Polish: English First 100 Words,...
Are you ready to embark on a linguistic...
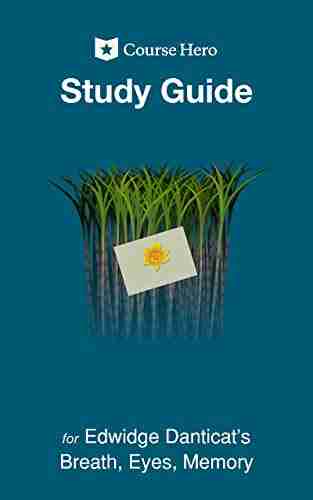

Unlock the Secrets of Edwidge Danticat's Breath, Eyes,...
Are you delving into the world...
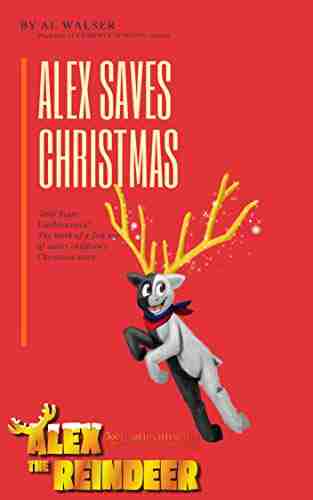

300 Years Liechtenstein: The Birth of Fish Out of Water...
Once upon a time, in the...
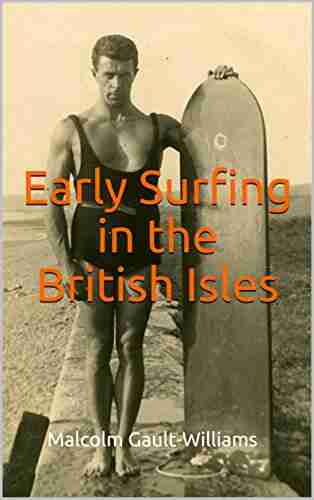

Exploring the Legendary Surfers of Early Surfing in the...
Surfing, a sport...
Light bulbAdvertise smarter! Our strategic ad space ensures maximum exposure. Reserve your spot today!
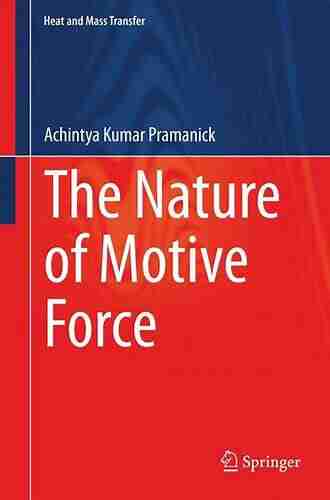

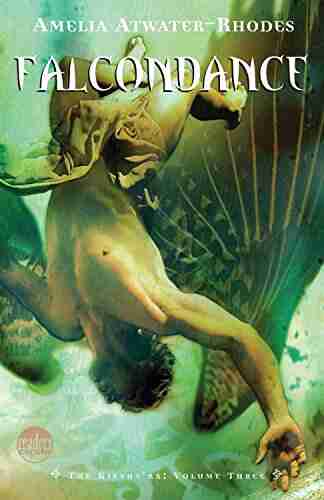

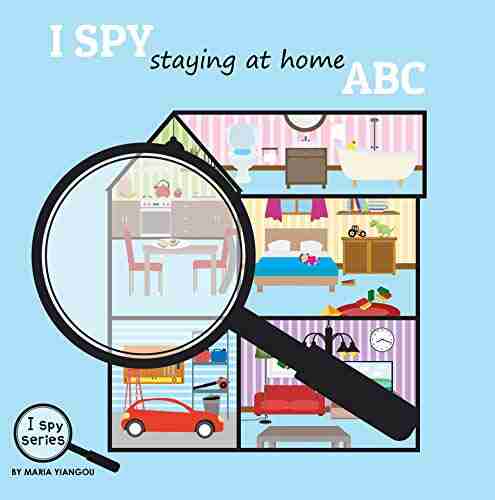

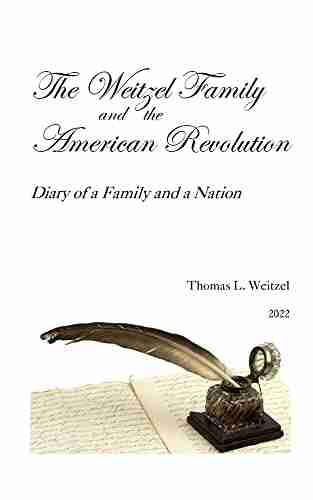

- Isaac BellFollow ·3.4k
- Kazuo IshiguroFollow ·17.3k
- Thomas PynchonFollow ·14.9k
- Cruz SimmonsFollow ·8.7k
- Russell MitchellFollow ·18.3k
- Carl WalkerFollow ·11.5k
- Dwight BellFollow ·7.7k
- Isaiah PriceFollow ·19.7k