Book Downloads Hub Reads Ebooks Online eBook Librarys Digital Books Store Download Book Pdfs Bookworm Downloads Free Books Downloads Epub Book Collection Pdf Book Vault Read and Download Books Open Source Book Library Best Book Downloads James Curcio Uladzislau Belavusau Josiah Quincy Joseph Black Ali Dean James Williams Tom Lansford Aidin Safavi
Do you want to contribute by writing guest posts on this blog?
Please contact us and send us a resume of previous articles that you have written.
Unlock the Power of Optimization Techniques in Statistics: Enhancing Statistical Modeling and Decision Science

Statistics, a branch of mathematics that deals with the collection, analysis, interpretation, presentation, and organization of data, plays a crucial role in various fields. It enables researchers and organizations to draw meaningful insights and make informed decisions. However, the real power of statistics lies in its optimization techniques, which can revolutionize statistical modeling and decision science.
What are Optimization Techniques?
Optimization techniques refer to a set of mathematical methods used to find the best solutions for complex problems. In statistics, these techniques help in maximizing or minimizing an objective function while satisfying certain constraints. They aim to improve efficiency, accuracy, and decision-making by optimizing various statistical models.
Optimization techniques in statistics can be broadly classified into two categories: deterministic and stochastic. Deterministic optimization involves finding the optimal solution using mathematical equations and algorithms. On the other hand, stochastic optimization utilizes probabilistic models and algorithms to find near-optimal solutions in situations where randomness is involved.
5 out of 5
Language | : | English |
File size | : | 25772 KB |
X-Ray for textbooks | : | Enabled |
Print length | : | 359 pages |
Screen Reader | : | Supported |
The Role of Optimization Techniques in Statistical Modeling
Statistical modeling involves developing mathematical equations and models to describe and predict the relationships between variables. Optimization techniques can significantly enhance the accuracy and efficiency of statistical models, leading to more precise predictions and interpretations.
Some commonly used optimization techniques in statistical modeling include:
- Linear Programming: Linear programming helps in optimizing linear equations subject to linear constraints. It finds applications in resource allocation, production planning, portfolio optimization, and transportation.
- Nonlinear Programming: Nonlinear programming deals with optimizing nonlinear equations. It is used to solve complex optimization problems in various fields, such as economics, engineering, and data analysis.
- Integer Programming: Integer programming involves finding optimal solutions to linear or nonlinear equations with integer variables. It is useful in decision-making processes that involve discrete choices, such as project selection, scheduling, and facility location.
- Convex Programming: Convex programming deals with optimizing convex objective functions and convex constraints. It finds applications in machine learning, data fitting, signal processing, and image reconstruction.
- Dynamic Programming: Dynamic programming breaks complex problems into smaller subproblems, solving them iteratively. It is particularly useful in decision-making problems involving sequential actions and uncertain environments.
Optimization Techniques in Decision Science
Decision science involves making informed decisions based on available data, statistical models, and analyses. Optimization techniques play a crucial role in decision science by providing tools to optimize decision-making processes and improve outcomes.
Some optimization techniques used in decision science include:
- Game Theory: Game theory employs mathematical models to analyze and optimize decision-making in competitive situations. It helps in determining optimal strategies for players, maximizing overall outcomes, and predicting behavior.
- Queueing Theory: Queueing theory involves analyzing and optimizing waiting lines or queues. It finds applications in service industries, transportation, telecommunication networks, and healthcare systems.
- Network Optimization: Network optimization focuses on finding the most efficient paths or flows in networks. It is used in logistics, transportation planning, telecommunication routing, and supply chain management.
- Simulation Optimization: Simulation optimization combines simulation modeling with optimization techniques to find the best solution for complex systems. It is used in risk analysis, investment planning, and process improvement.
The Advantages of Optimization Techniques in Statistics and Decision Making
Optimization techniques offer numerous benefits in both statistical modeling and decision science:
- Improved Efficiency: By finding optimal solutions, optimization techniques enhance the efficiency of statistical models and decision-making processes. This leads to resource utilization, time management, and cost optimization.
- Enhanced Accuracy: Optimization techniques allow statisticians and decision-makers to make more accurate predictions and draw precise s. This, in turn, helps in minimizing errors and uncertainty.
- Robust Decision-Making: Optimization techniques enable the evaluation of multiple scenarios, trade-offs, and constraints to ensure robust decision-making. They provide insights into the potential outcomes of different options and help in selecting the most optimal course of action.
- Real-Time Decision Support: Using optimization techniques, decisions can be made in real-time, taking into account changing circumstances, constraints, and objectives. This enables organizations to respond quickly to dynamic environments.
- Competitive Advantage: Implementing optimization techniques gives organizations a competitive edge by streamlining processes, improving efficiency, and making better-informed decisions. This can lead to higher profitability, growth, and customer satisfaction.
Optimization techniques in statistics have the potential to revolutionize statistical modeling and decision science. They offer a wide range of methods to optimize objective functions, improve efficiency, and enhance decision-making processes. By embracing these techniques, researchers and organizations can unlock the power of data, making better-informed decisions and gaining a competitive advantage.
5 out of 5
Language | : | English |
File size | : | 25772 KB |
X-Ray for textbooks | : | Enabled |
Print length | : | 359 pages |
Screen Reader | : | Supported |
Statistics help guide us to optimal decisions under uncertainty. A large variety of statistical problems are essentially solutions to optimization problems. The mathematical techniques of optimization are fundamentalto statistical theory and practice. In this book, Jagdish Rustagi provides full-spectrum coverage of these methods, ranging from classical optimization and Lagrange multipliers, to numerical techniques using gradients or direct search, to linear, nonlinear, and dynamic programming using the Kuhn-Tucker conditions or the Pontryagin maximal principle. Variational methods and optimization in function spaces are also discussed, as are stochastic optimization in simulation, including annealing methods.
The text features numerous applications, including: Finding maximum likelihood estimates, Markov decision processes, Programming methods used to optimize monitoring of patients in hospitals, Derivation of the Neyman-Pearson lemma, The search for optimal designs, Simulation of a steel mill.
Suitable as both a reference and a text, this book will be of interest to advanced undergraduate or beginning graduate students in statistics, operations research, management and engineering sciences, and related fields. Most of the material can be covered in one semester by students with a basic background in probability and statistics.
- Covers optimization from traditional methods to recent developments such as Karmarkars algorithm and simulated annealing
- Develops a wide range of statistical techniques in the unified context of optimization
- Discusses applications such as optimizing monitoring of patients and simulating steel mill operations
- Treats numerical methods and applications
- Includes exercises and references for each chapter
- Covers topics such as linear, nonlinear, and dynamic programming, variational methods, and stochastic optimization
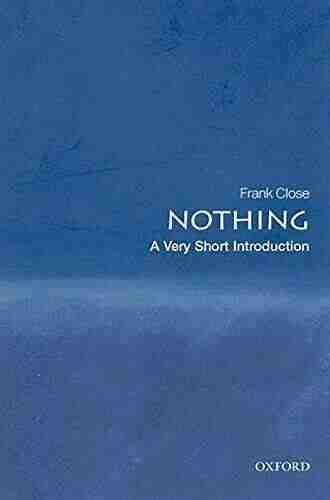

The Most Insightful and Liberating Experiences Found in...
When it comes to expanding our...
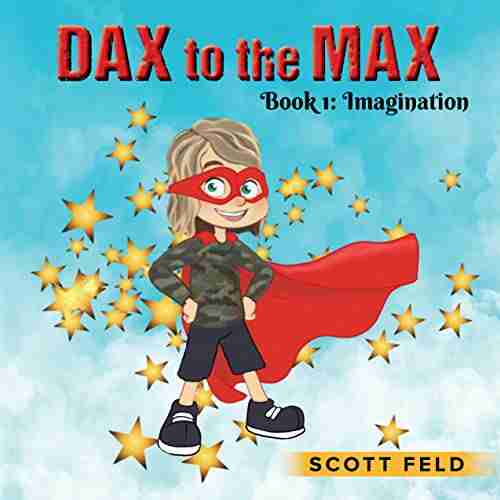

Dax To The Max Imagination: Unlock the Power of...
Welcome to the world of Dax To...
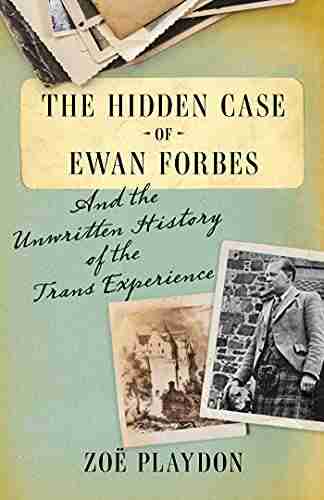

The Hidden Case of Ewan Forbes: Uncovering the Mystery...
Ewan Forbes: a...
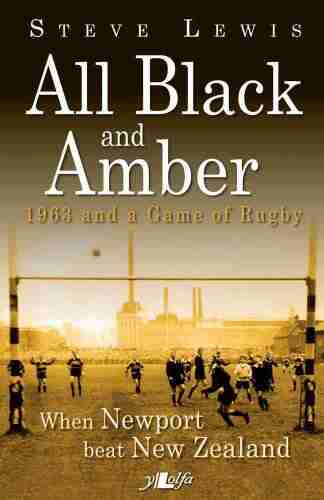

When Newport Beat New Zealand: A Historic Rugby Upset
The rivalry between Newport and New Zealand...
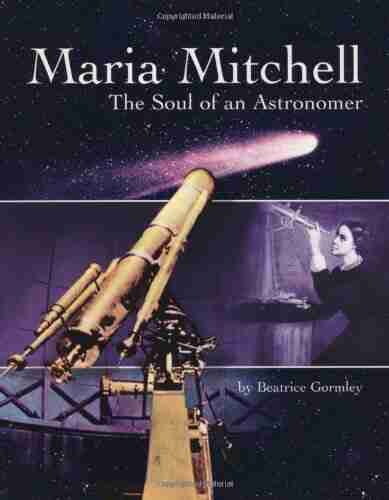

The Soul of an Astronomer: Women of Spirit
Astronomy, the study of...
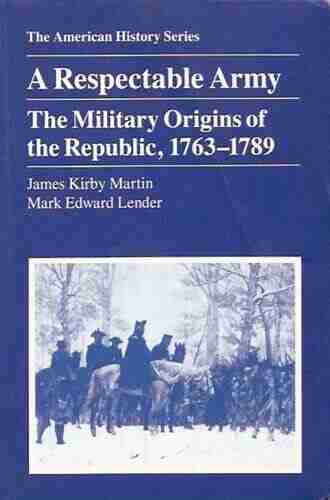

The Military Origins Of The Republic 1763-1789
When we think about the birth of the...
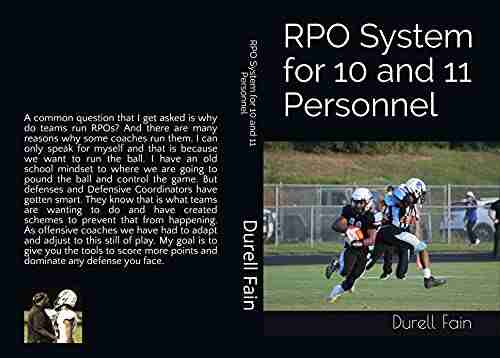

RPO System for 10 and 11 Personnel: Durell Fain
When it comes to...
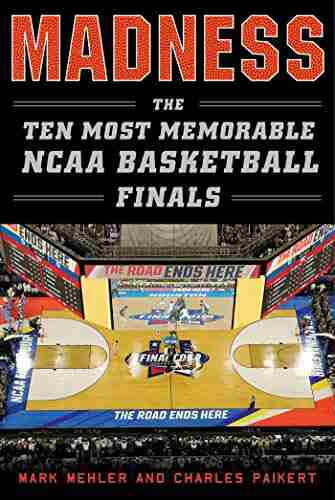

Madness: The Ten Most Memorable NCAA Basketball Finals
College basketball fans eagerly await the...
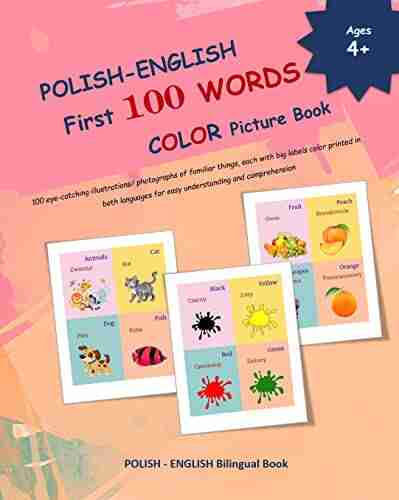

Discover the Magic of Polish: English First 100 Words,...
Are you ready to embark on a linguistic...
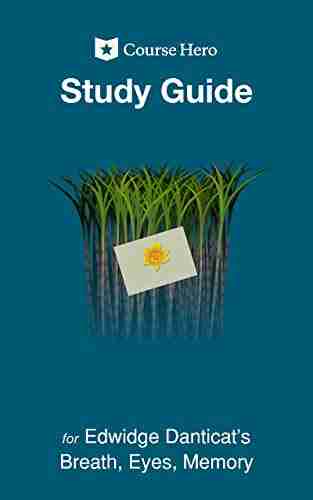

Unlock the Secrets of Edwidge Danticat's Breath, Eyes,...
Are you delving into the world...
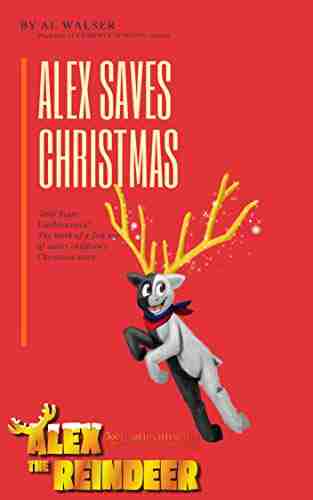

300 Years Liechtenstein: The Birth of Fish Out of Water...
Once upon a time, in the...
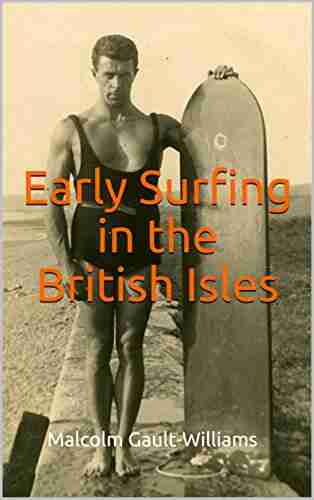

Exploring the Legendary Surfers of Early Surfing in the...
Surfing, a sport...
Light bulbAdvertise smarter! Our strategic ad space ensures maximum exposure. Reserve your spot today!
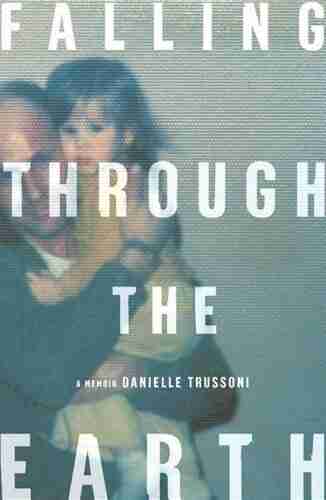

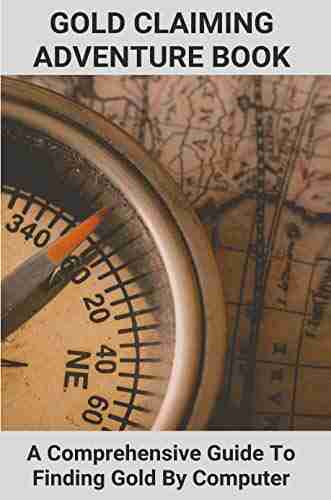

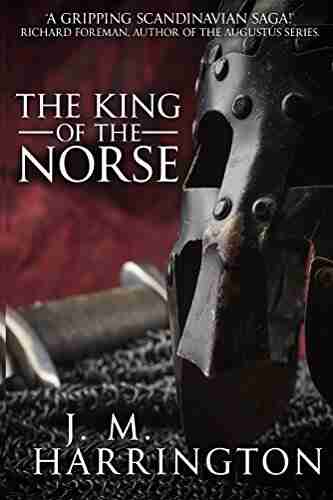

- Levi PowellFollow ·6.1k
- Marcel ProustFollow ·12.6k
- Dwight BellFollow ·7.7k
- Charles BukowskiFollow ·2.2k
- Gabriel BlairFollow ·7.5k
- Anthony BurgessFollow ·7.6k
- Joseph ConradFollow ·15.5k
- Duncan CoxFollow ·4.6k