Book Downloads Hub Reads Ebooks Online eBook Librarys Digital Books Store Download Book Pdfs Bookworm Downloads Free Books Downloads Epub Book Collection Pdf Book Vault Read and Download Books Open Source Book Library Best Book Downloads Jana Deleon Marjorie Herrera Lewis Thomas More Codex Regius Roland Smith Carolyn Steele Ait Outoulboun Essaid Maria Yiangou
Do you want to contribute by writing guest posts on this blog?
Please contact us and send us a resume of previous articles that you have written.
Understanding Nonstationarities in Hydrologic and Environmental Time Series Data

Welcome to the fascinating world of hydrology and environmental science, where the forces of nature shape our planet and dictate the availability of essential resources like water. In this article, we will explore the concept of nonstationarities in hydrologic and environmental time series data, unraveling the hidden complexities that lie beneath the surface of these seemingly regular observations.
What are Nonstationarities?
To understand nonstationarities, let's first grasp the concept of stationarity. In time series analysis, a process is said to be stationary if its statistical properties, such as mean and variance, remain constant over time. By assuming stationarity, scientists are able to make reliable predictions based on historical data. However, in the real world, this assumption rarely holds true.
Nonstationarities refer to the violations of stationarity assumptions, especially in hydrologic and environmental time series data. These nonstationarities can manifest themselves in various forms:
4.7 out of 5
Language | : | English |
File size | : | 9494 KB |
Text-to-Speech | : | Enabled |
Screen Reader | : | Supported |
Word Wise | : | Enabled |
Print length | : | 392 pages |
- Trends: Long-term changes in the mean value of the time series, either increasing or decreasing over time.
- Seasonality: Regular patterns in the time series that repeat at fixed intervals, such as daily, monthly, or annual cycles.
- Cycles: Longer-term patterns in the time series that occur over irregular intervals, such as the El Niño Southern Oscillation (ENSO) cycle.
- Structural Breaks: Sudden shifts in the statistical properties of the time series, often caused by external events or policy changes.
- Autocorrelation: Dependence between observations in the time series, where the value of a particular observation is influenced by previous observations.
The Implications of Nonstationarities
Nonstationarities can have profound implications for water scientists and environmental researchers. By neglecting these complexities and assuming stationarity, our predictions and decision-making processes may yield inaccurate results.
For example, consider a hydrologist responsible for predicting river flows. If the underlying trend of increasing flows is not accounted for, the predictions might lead water managers to build dams or irrigation systems that are insufficient to handle future demands. Conversely, ignoring a decreasing trend could result in overestimating reservoir capacities and wasting valuable resources.
Similarly, nonstationarities in environmental time series data can have significant impacts. Climate change-induced trends and shifts in seasonal patterns can affect agricultural productivity, biodiversity, and the spread of diseases. Failing to acknowledge these nonstationarities hinders our ability to develop effective mitigation and adaptation strategies.
Methods for Detecting Nonstationarities
Thankfully, modern statistical methods and data analysis techniques allow us to identify and analyze nonstationarities in hydrologic and environmental time series data. Some commonly used methods include:
- Time Series Decomposition: Separating a time series into its trend, seasonal, and residual components to reveal underlying patterns and nonstationarities.
- Autocorrelation Analysis: Assessing the correlation between observations at different lags to detect dependencies and potential structural breaks.
- Wavelet Analysis: Decomposing a time series into different frequency components to identify time-varying trends and cyclical behaviors.
- Change Point Detection: Identifying abrupt changes in the statistical properties of a time series using algorithms and statistical tests.
- Machine Learning Techniques: Utilizing advanced algorithms to capture and model nonstationarities based on historical patterns and observed data.
By employing these methods, researchers can gain insights into the underlying processes causing nonstationarities and develop more accurate models for future predictions and decision-making.
The Role of Nonstationarities in Water Resources Management
Water resources management is a field heavily impacted by nonstationarities. As the demand for water continues to rise due to population growth and climate change, understanding and accounting for nonstationarities becomes paramount in ensuring sustainable water management strategies.
Studies have shown that nonstationarities in hydrologic time series data can lead to significant errors in water resource planning and management. By incorporating nonstationarities through advanced modeling techniques and considering future climate scenarios, water managers can better assess the risks associated with extreme events like floods and droughts, optimize water allocation and storage, and design infrastructure resilient to changing conditions.
, nonstationarities pose challenges and opportunities in the field of hydrologic and environmental science. By acknowledging the presence of nonstationarities in time series data and employing appropriate analysis methods, we can unlock valuable insights into the changing dynamics of our natural systems and make informed decisions to ensure a sustainable future.
So let's dive deeper, explore the intricacies of nonstationarities, and unravel the mysteries that lie within the vast realm of hydrologic and environmental time series data.
4.7 out of 5
Language | : | English |
File size | : | 9494 KB |
Text-to-Speech | : | Enabled |
Screen Reader | : | Supported |
Word Wise | : | Enabled |
Print length | : | 392 pages |
Conventionally, time series have been studied either in the time domain or the frequency domain. The representation of a signal in the time domain is localized in time, i.e . the value of the signal at each instant in time is well defined . However, the time representation of a signal is poorly localized in frequency , i.e. little information about the frequency content of the signal at a certain frequency can be known by looking at the signal in the time domain . On the other hand, the representation of a signal in the frequency domain is well localized in frequency, but is poorly localized in time, and as a consequence it is impossible to tell when certain events occurred in time. In studying stationary or conditionally stationary processes with mixed spectra , the separate use of time domain and frequency domain analyses is sufficient to reveal the structure of the process . Results discussed in the previous chapters suggest that the time series analyzed in this book are conditionally stationary processes with mixed spectra. Additionally, there is some indication of nonstationarity, especially in longer time series.
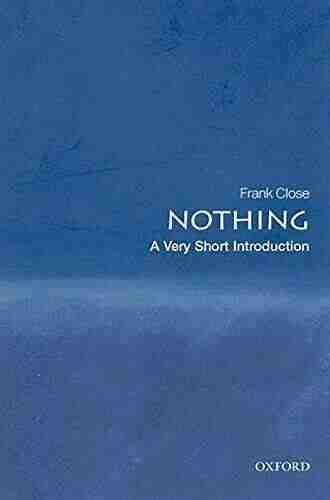

The Most Insightful and Liberating Experiences Found in...
When it comes to expanding our...
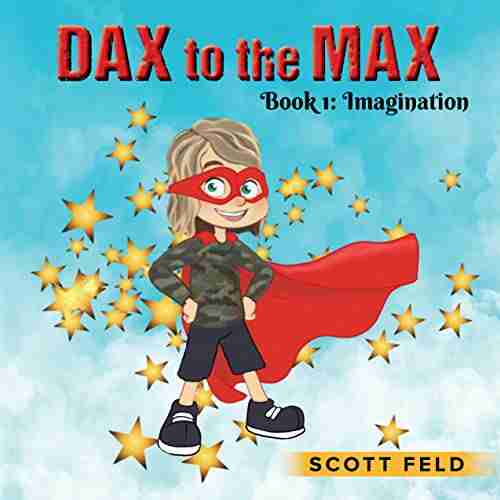

Dax To The Max Imagination: Unlock the Power of...
Welcome to the world of Dax To...
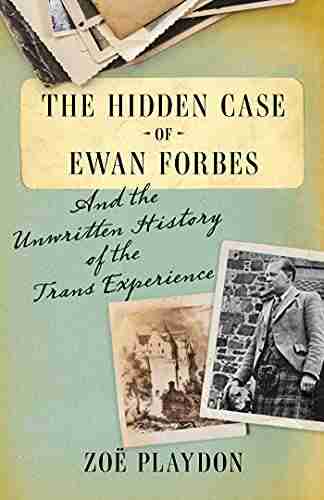

The Hidden Case of Ewan Forbes: Uncovering the Mystery...
Ewan Forbes: a...
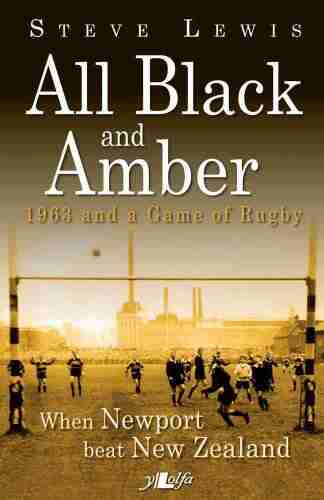

When Newport Beat New Zealand: A Historic Rugby Upset
The rivalry between Newport and New Zealand...
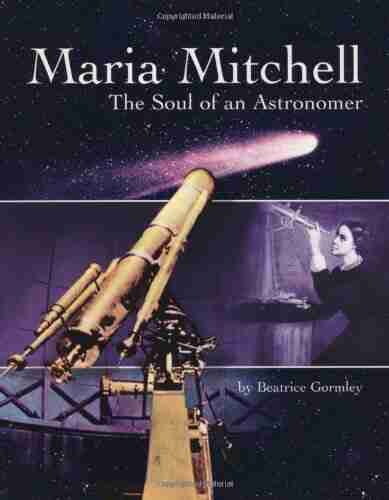

The Soul of an Astronomer: Women of Spirit
Astronomy, the study of...
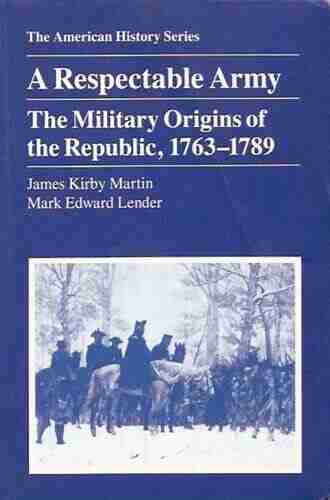

The Military Origins Of The Republic 1763-1789
When we think about the birth of the...
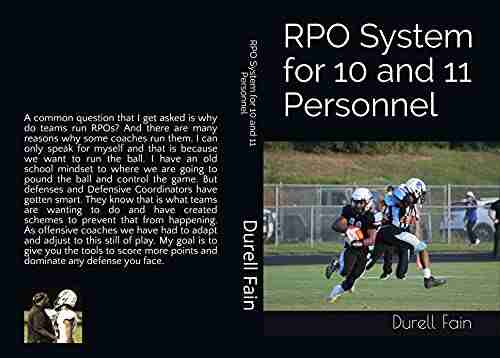

RPO System for 10 and 11 Personnel: Durell Fain
When it comes to...
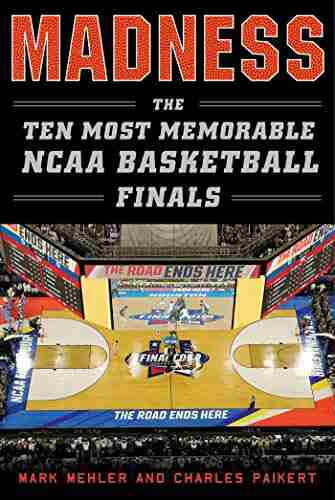

Madness: The Ten Most Memorable NCAA Basketball Finals
College basketball fans eagerly await the...
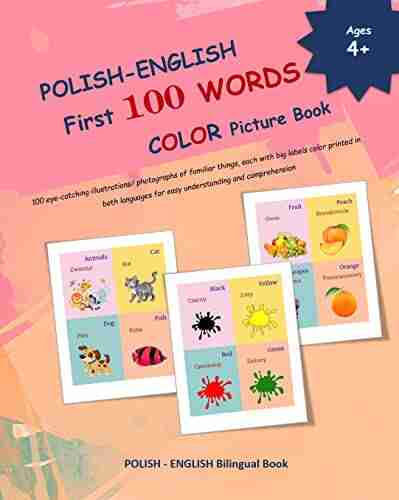

Discover the Magic of Polish: English First 100 Words,...
Are you ready to embark on a linguistic...
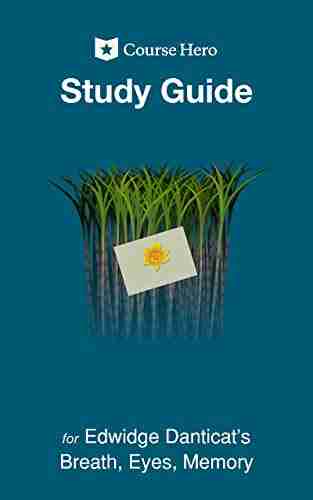

Unlock the Secrets of Edwidge Danticat's Breath, Eyes,...
Are you delving into the world...
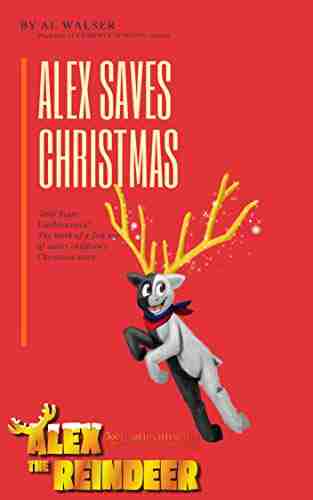

300 Years Liechtenstein: The Birth of Fish Out of Water...
Once upon a time, in the...
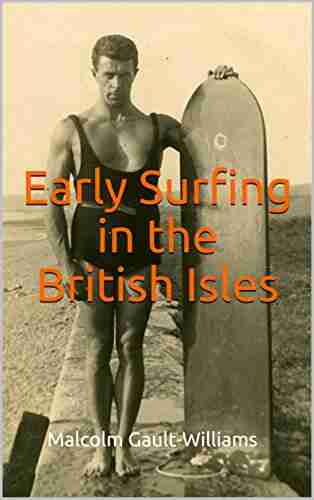

Exploring the Legendary Surfers of Early Surfing in the...
Surfing, a sport...
Light bulbAdvertise smarter! Our strategic ad space ensures maximum exposure. Reserve your spot today!
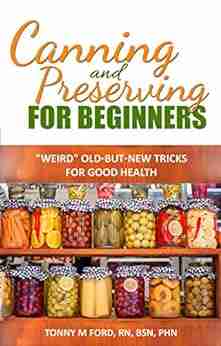

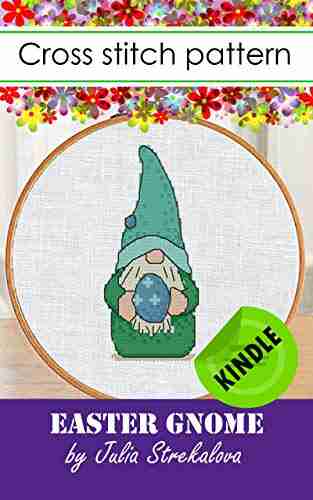

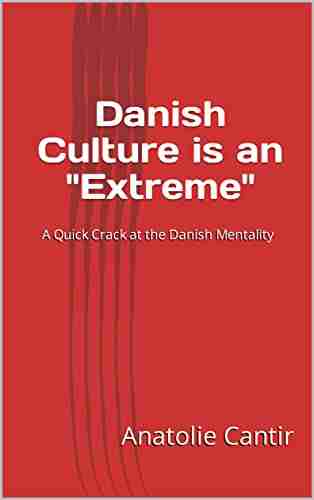

- E.E. CummingsFollow ·19.9k
- Graham BlairFollow ·14.5k
- Gary CoxFollow ·16.7k
- Mitch FosterFollow ·16k
- Thomas PowellFollow ·4.5k
- Ken FollettFollow ·2.2k
- Morris CarterFollow ·15.5k
- J.D. SalingerFollow ·3.5k