Book Downloads Hub Reads Ebooks Online eBook Librarys Digital Books Store Download Book Pdfs Bookworm Downloads Free Books Downloads Epub Book Collection Pdf Book Vault Read and Download Books Open Source Book Library Best Book Downloads Geoff Canham David Horowitz Jonathan E Schroeder Richard Jefferies Jodi Tyler Sophie Kirtley Stephen Chambers Tricia Frey
Do you want to contribute by writing guest posts on this blog?
Please contact us and send us a resume of previous articles that you have written.
Machine Learning For Data Streams - Revolutionizing Real-Time Data Analysis

In today's digital age, we are generating vast amounts of data every single day. From social media interactions to online shopping habits, our activities leave behind digital footprints that can be analyzed to gain valuable insights. However, with the sheer volume and velocity of data being generated, traditional machine learning algorithms often struggle to keep up. This is where machine learning for data streams comes into play - revolutionizing real-time data analysis.
The Challenges of Analyzing Data Streams
Data streams refer to continuous, rapidly arriving data that needs to be processed and analyzed in real-time. This includes data from various sources such as online transactions, sensor readings, social media feeds, and more. The primary challenge with data streams is their dynamic nature - they evolve and change over time, making it difficult to build static models that can accurately predict outcomes.
Furthermore, data streams often have high data velocities, with new data points arriving at a rapid rate. Traditional machine learning algorithms are not designed to handle such high-speed data streams, as they typically assume that data is available all at once for training and model building.
4.1 out of 5
Language | : | English |
File size | : | 20296 KB |
Text-to-Speech | : | Enabled |
Screen Reader | : | Supported |
Enhanced typesetting | : | Enabled |
Print length | : | 355 pages |
Paperback | : | 194 pages |
Item Weight | : | 10.2 ounces |
Dimensions | : | 6 x 0.44 x 9 inches |
The Need for Machine Learning in Real-Time
Real-time data analysis is crucial in today's fast-paced world. Organizations across industries rely on real-time insights to make informed decisions and respond quickly to changing market conditions. Machine learning algorithms that can handle data streams provide the necessary framework for analyzing data in real-time, enabling businesses to gain competitive advantages.
Advantages of Machine Learning for Data Streams
Machine learning for data streams offers several advantages over traditional approaches. Firstly, it allows for continuous learning, meaning models can adapt to changing patterns and trends in real-time. This ensures that predictions and recommendations remain accurate and up-to-date.
Secondly, machine learning algorithms designed for data streams are typically memory-efficient. They do not require large amounts of storage to maintain historical data, making them suitable for high-velocity data streams with limited resources.
Another advantage is their ability to handle concept drift. Concept drift refers to the phenomenon where the statistical properties of the data change over time. This is often encountered in data streams, as new features or patterns emerge, rendering existing models ineffective. Machine learning algorithms for data streams can dynamically update their models to adapt to these changes, ensuring continued accuracy in predictions.
Applications of Machine Learning for Data Streams
The applications of machine learning for data streams are wide-ranging. In finance, real-time analysis of stock market data streams can help traders make better investment decisions. In healthcare, real-time monitoring of patient data can assist in early detection of critical conditions. In e-commerce, real-time analysis of customer interactions can enable personalized recommendations and improve customer satisfaction.
Furthermore, machine learning for data streams is also crucial in cybersecurity. Real-time analysis of network traffic and user behavior can help detect and prevent cyberattacks. Similarly, in transportation and logistics, real-time analysis of sensor data can optimize routes and predict maintenance needs.
Machine learning for data streams represents a significant advancement in real-time data analysis. It allows organizations to make informed decisions quickly and respond effectively to ever-changing market dynamics. With continuous learning, memory-efficiency, and the ability to handle concept drift, machine learning algorithms designed for data streams are revolutionizing the way we analyze and harness the power of real-time data. In today's data-driven world, embracing machine learning for data streams is essential for staying ahead of the competition and unlocking valuable insights hidden within the streams of data flowing around us.
4.1 out of 5
Language | : | English |
File size | : | 20296 KB |
Text-to-Speech | : | Enabled |
Screen Reader | : | Supported |
Enhanced typesetting | : | Enabled |
Print length | : | 355 pages |
Paperback | : | 194 pages |
Item Weight | : | 10.2 ounces |
Dimensions | : | 6 x 0.44 x 9 inches |
A hands-on approach to tasks and techniques in data stream mining and real-time analytics, with examples in MOA, a popular freely available open-source software framework.
Today many information sources—including sensor networks, financial markets, social networks, and healthcare monitoring—are so-called data streams, arriving sequentially and at high speed. Analysis must take place in real time, with partial data and without the capacity to store the entire data set. This book presents algorithms and techniques used in data stream mining and real-time analytics. Taking a hands-on approach, the book demonstrates the techniques using MOA (Massive Online Analysis),a popular, freely available open-source software framework, allowing readers to try out the techniques after reading the explanations.
The book first offers a brief to the topic, covering big data mining, basic methodologies for mining data streams, and a simple example of MOA. More detailed discussions follow, with chapters on sketching techniques, change, classification, ensemble methods, regression, clustering, and frequent pattern mining. Most of these chapters include exercises, an MOA-based lab session, or both. Finally, the book discusses the MOA software, covering the MOA graphical user interface, the command line, use of its API, and the development of new methods within MOA. The book will be an essential reference for readers who want to use data stream mining as a tool, researchers in innovation or data stream mining, and programmers who want to create new algorithms for MOA.
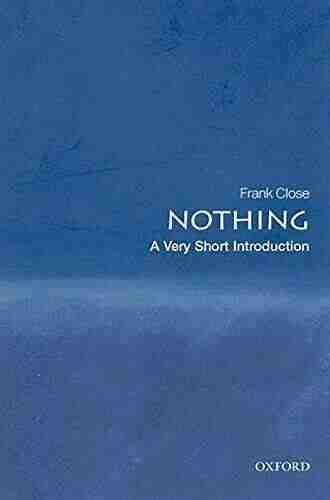

The Most Insightful and Liberating Experiences Found in...
When it comes to expanding our...
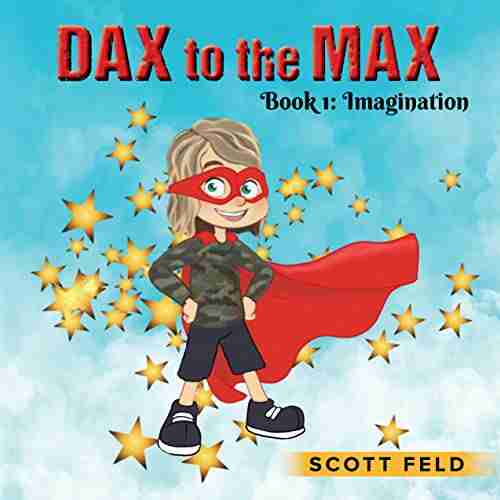

Dax To The Max Imagination: Unlock the Power of...
Welcome to the world of Dax To...
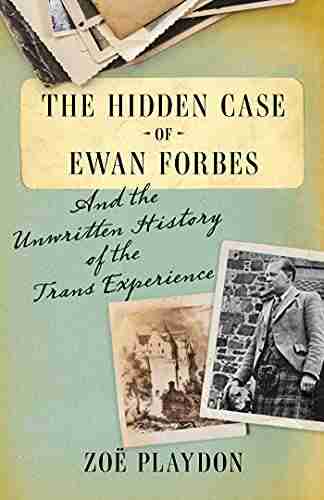

The Hidden Case of Ewan Forbes: Uncovering the Mystery...
Ewan Forbes: a...
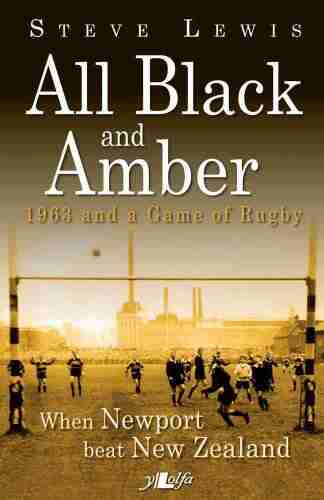

When Newport Beat New Zealand: A Historic Rugby Upset
The rivalry between Newport and New Zealand...
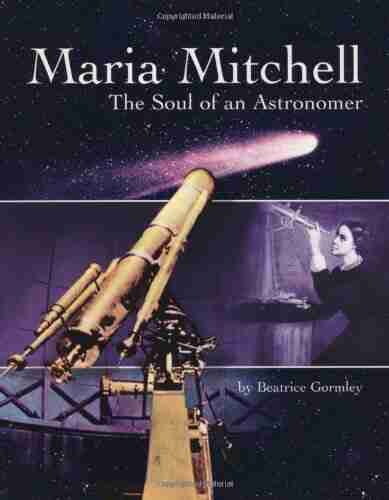

The Soul of an Astronomer: Women of Spirit
Astronomy, the study of...
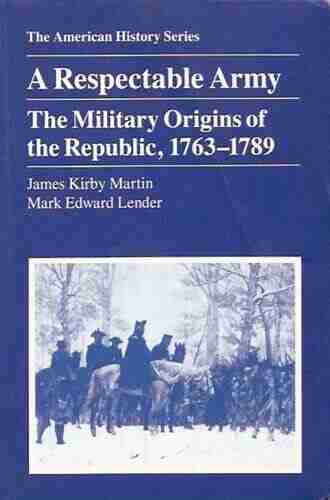

The Military Origins Of The Republic 1763-1789
When we think about the birth of the...
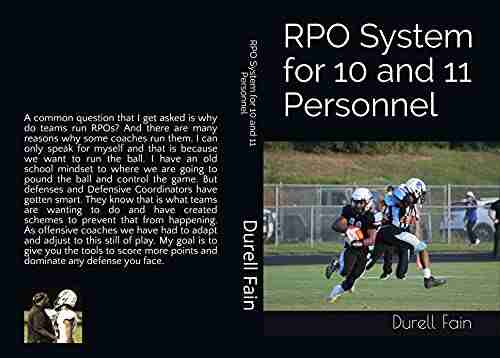

RPO System for 10 and 11 Personnel: Durell Fain
When it comes to...
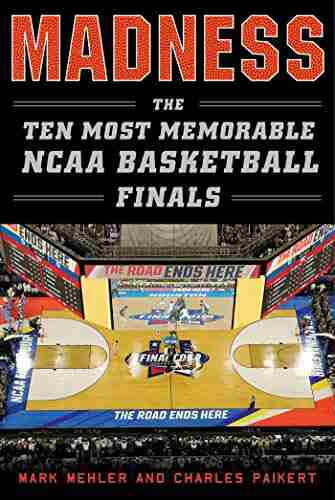

Madness: The Ten Most Memorable NCAA Basketball Finals
College basketball fans eagerly await the...
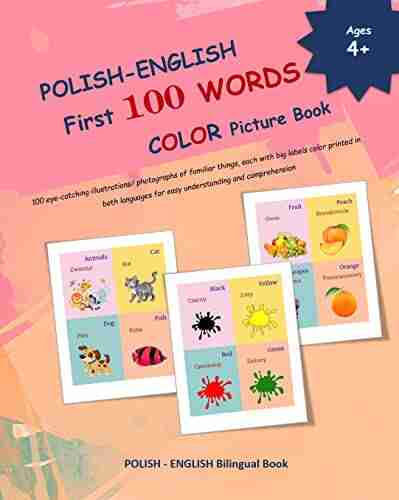

Discover the Magic of Polish: English First 100 Words,...
Are you ready to embark on a linguistic...
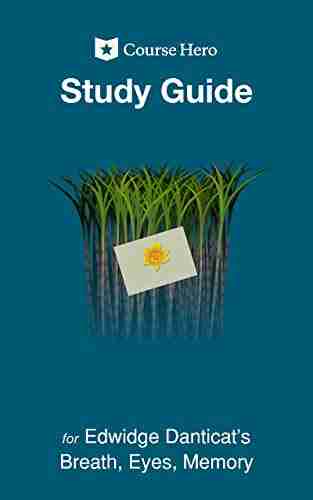

Unlock the Secrets of Edwidge Danticat's Breath, Eyes,...
Are you delving into the world...
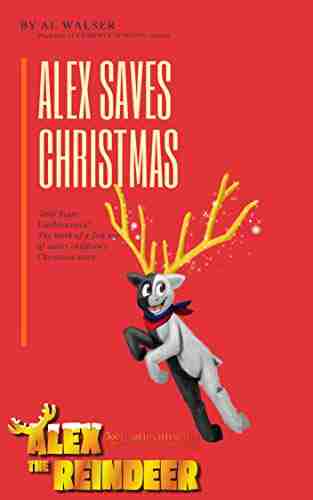

300 Years Liechtenstein: The Birth of Fish Out of Water...
Once upon a time, in the...
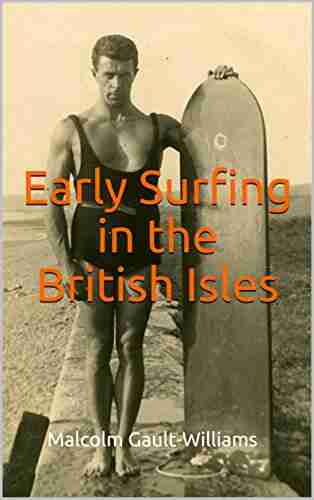

Exploring the Legendary Surfers of Early Surfing in the...
Surfing, a sport...
Light bulbAdvertise smarter! Our strategic ad space ensures maximum exposure. Reserve your spot today!
- Donald WardFollow ·11.5k
- Anton ChekhovFollow ·15k
- Federico García LorcaFollow ·7.2k
- Aubrey BlairFollow ·11.9k
- Danny SimmonsFollow ·16.9k
- Herbert CoxFollow ·11.2k
- Ivan TurgenevFollow ·13.1k
- Clarence MitchellFollow ·3.3k