Book Downloads Hub Reads Ebooks Online eBook Librarys Digital Books Store Download Book Pdfs Bookworm Downloads Free Books Downloads Epub Book Collection Pdf Book Vault Read and Download Books Open Source Book Library Best Book Downloads Sean Laidlaw Michael Putzel Rebecca Cunningham Emmanuel Mazer Jeffery Faulkerson Emily J Dolbear Michael Norman Adolph Barr
Do you want to contribute by writing guest posts on this blog?
Please contact us and send us a resume of previous articles that you have written.
Unveiling the Ultimate Solution: Automatic Defense Against Zero Day Polymorphic Worms In Communication Networks

Communication networks have become an integral part of our daily lives, connecting people, businesses, and devices across the globe. However, with every advancement in technology comes new challenges, and one of the most menacing threats to communication networks is the emergence of zero-day polymorphic worms.
Zero-day polymorphic worms are a type of malware that exploit previously unknown vulnerabilities in software, making them extremely difficult to detect and defend against. These worms can spread rapidly through communication networks, infecting a large number of devices within a short period of time. The consequences of such infections can be catastrophic, leading to data breaches, network disruptions, and financial losses.
Traditionally, defending against zero-day polymorphic worms has been a complex and time-consuming task. Security experts would need to analyze the worm's behavior, reverse-engineer its code, and develop countermeasures to mitigate the threat. However, this process can take days, if not weeks, during which the worm can continue to wreak havoc.
4.6 out of 5
Language | : | English |
File size | : | 22193 KB |
Print length | : | 337 pages |
Screen Reader | : | Supported |
Fortunately, technological advancements have paved the way for automatic defense mechanisms that can rapidly respond to zero-day polymorphic worms. These intelligent systems leverage machine learning algorithms to detect and neutralize malware in real-time, protecting communication networks from unprecedented threats.
Towards Automatic Defense: The Role of Machine Learning
Machine learning algorithms have proven to be a game-changer in the field of cybersecurity. These algorithms can analyze large volumes of data, identify patterns, and make predictions based on historical information. By training these algorithms on vast datasets containing information about known malware, it becomes possible to detect and classify new strains of polymorphic worms.
The process begins by collecting data about network traffic, system logs, and behavior patterns of users and devices. This data is then fed into the machine learning system, which undergoes a training phase where it learns to distinguish normal network behavior from malicious activity.
During the training phase, the machine learning system identifies various features that are indicative of polymorphic worms. These features include but are not limited to unusual network traffic spikes, abnormal CPU or memory usage, and suspicious file access patterns.
Once the machine learning system completes its training, it enters the active defense mode. In this mode, it continuously monitors the network, analyzing incoming and outgoing traffic in real-time. As soon as the system detects any anomalous behavior that matches the patterns of a polymorphic worm, it triggers a response to neutralize the threat.
Common response measures include blocking network connections associated with the worm, alerting network administrators, or executing mitigation strategies to contain the spread of the worm. These actions are performed automatically, without requiring human intervention, therefore drastically reducing the response time and minimizing the potential damage caused by the worm.
Benefits and Limitations of Automatic Defense Mechanisms
Automatic defense mechanisms offer numerous benefits in dealing with zero-day polymorphic worms. Firstly, these systems can detect and respond to previously unknown strains of malware, providing proactive protection against evolving threats. Secondly, the ability to automatically counteract worms minimizes the risk of human error and ensures swift response times, preventing the worm from spreading further.
Furthermore, machine learning-based defense systems can learn and adapt over time, improving their effectiveness in detecting and defending against ever-changing malware variants. As more data is collected and analyzed, these systems become more accurate and efficient, making them a vital component in the fight against zero-day polymorphic worms.
However, it is important to acknowledge that automatic defense mechanisms are not foolproof and have their limitations. Machine learning algorithms heavily rely on the quality and relevance of the training data. If the training data does not accurately represent the diverse range of potential threats, the system may fail to detect new variants of polymorphic worms.
Moreover, sophisticated attackers can employ advanced techniques to evade detection by automatic defense systems. These techniques may involve obfuscation, encryption, or dynamic behavior modification, making it challenging to identify polymorphic worms. As cybercriminals continue to innovate, it becomes crucial to continuously enhance and evolve automatic defense systems to maintain their effectiveness.
The Future of Automatic Defense Against Zero Day Polymorphic Worms
The battle against zero-day polymorphic worms in communication networks is an ongoing struggle, with both attackers and defenders constantly pushing the boundaries of innovation and technology. Automatic defense mechanisms powered by machine learning have emerged as a promising solution, providing real-time protection and enabling networks to stay one step ahead of polymorphic worms.
As we move forward, further advancements in machine learning, artificial intelligence, and big data analytics will strengthen automatic defense systems. These technologies will enable faster and more accurate detection of polymorphic worms, while also improving the ability to respond swiftly and decisively.
Additionally, collaborations between cybersecurity experts, researchers, and industry leaders are essential to staying ahead of the ever-evolving threat landscape. Sharing knowledge, exchanging insights, and engaging in continuous research will pave the way for more robust and resilient defense mechanisms against polymorphic worms.
, the emergence of zero-day polymorphic worms poses a significant challenge to communication networks. However, with automatic defense mechanisms powered by machine learning, we can effectively combat these threats. By continually evolving and adapting our defense strategies, we can ensure the integrity and security of our communication networks, safeguarding our digital world.
4.6 out of 5
Language | : | English |
File size | : | 22193 KB |
Print length | : | 337 pages |
Screen Reader | : | Supported |
Able to propagate quickly and change their payload with each infection, polymorphic worms have been able to evade even the most advanced intrusion detection systems (IDS). And, because zero-day worms require only seconds to launch flooding attacks on your servers, using traditional methods such as manually creating and storing signatures to defend against these threats is just too slow.
Bringing together critical knowledge and research on the subject, Automatic Defense Against Zero-day Polymorphic Worms in Communication Networks details a new approach for generating automated signatures for unknown polymorphic worms. It presents experimental results on a new method for polymorphic worm detection and examines experimental implementation of signature-generation algorithms and double-honeynet systems.
If you need some background, the book includes an overview of the fundamental terms and concepts in network security, including the various security models. Clearing up the misconceptions about the value of honeypots, it explains how they can be useful in securing your networks, and identifies open-source tools you can use to create your own honeypot. There’s also a chapter with references to helpful reading resources on automated signature generation systems.
The authors describe cutting-edge attack detection approaches and detail new algorithms to help you generate your own automated signatures for polymorphic worms. Explaining how to test the quality of your generated signatures, the text will help you develop the understanding required to effectively protect your communication networks. Coverage includes intrusion detection and prevention systems (IDPS),zero-day polymorphic worm collection methods, double-honeynet system configurations, and the implementation of double-honeynet architectures.
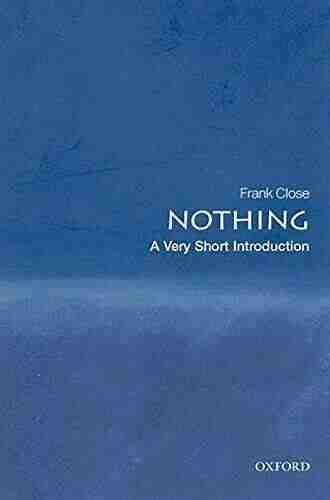

The Most Insightful and Liberating Experiences Found in...
When it comes to expanding our...
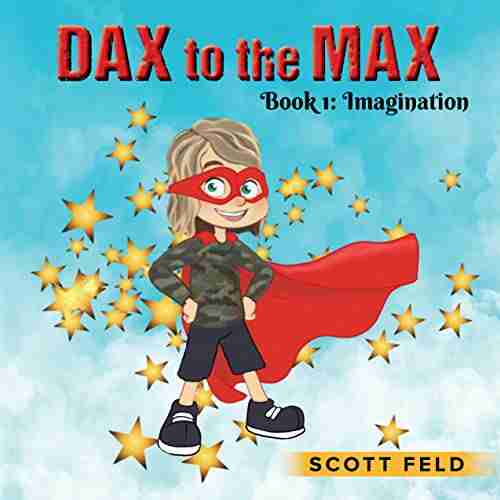

Dax To The Max Imagination: Unlock the Power of...
Welcome to the world of Dax To...
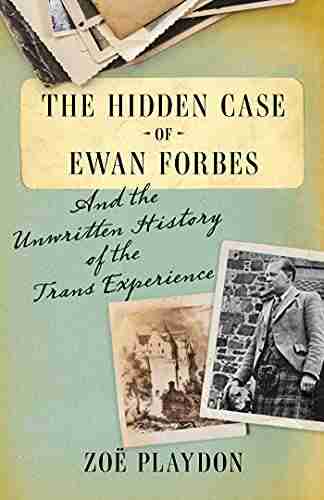

The Hidden Case of Ewan Forbes: Uncovering the Mystery...
Ewan Forbes: a...
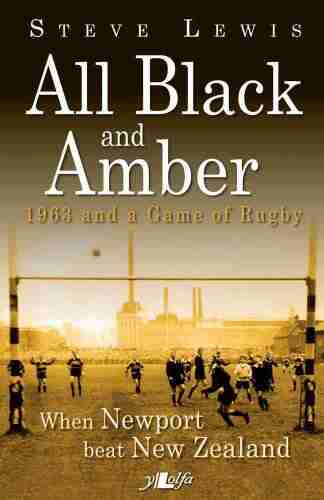

When Newport Beat New Zealand: A Historic Rugby Upset
The rivalry between Newport and New Zealand...
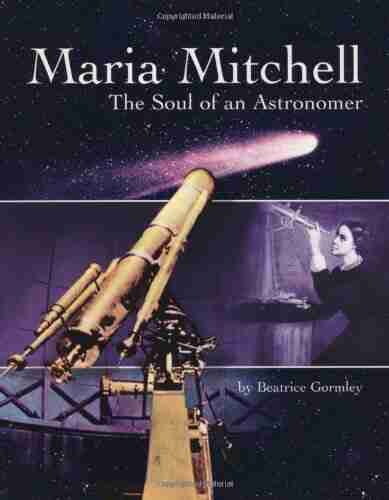

The Soul of an Astronomer: Women of Spirit
Astronomy, the study of...
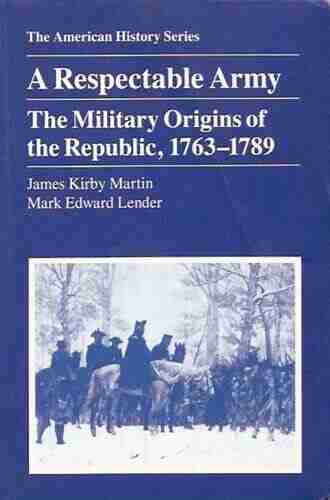

The Military Origins Of The Republic 1763-1789
When we think about the birth of the...
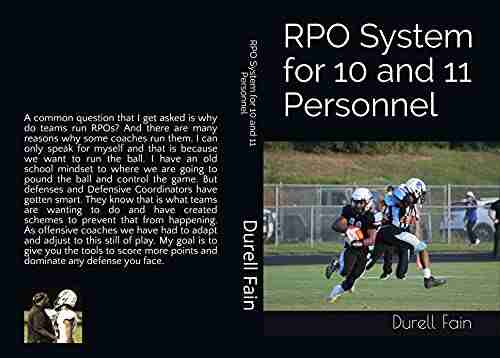

RPO System for 10 and 11 Personnel: Durell Fain
When it comes to...
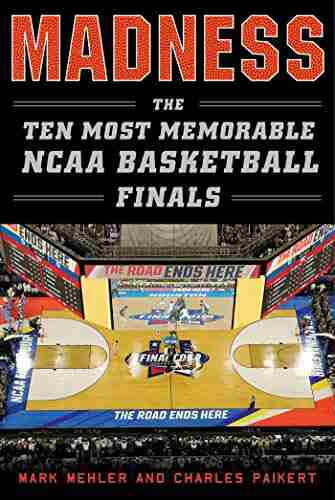

Madness: The Ten Most Memorable NCAA Basketball Finals
College basketball fans eagerly await the...
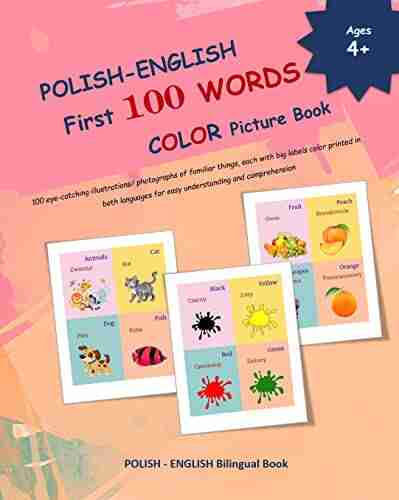

Discover the Magic of Polish: English First 100 Words,...
Are you ready to embark on a linguistic...
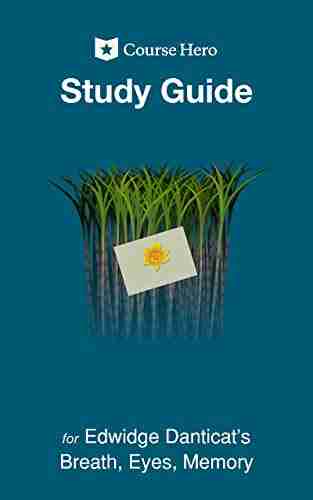

Unlock the Secrets of Edwidge Danticat's Breath, Eyes,...
Are you delving into the world...
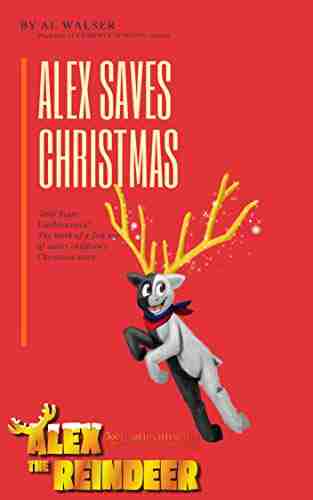

300 Years Liechtenstein: The Birth of Fish Out of Water...
Once upon a time, in the...
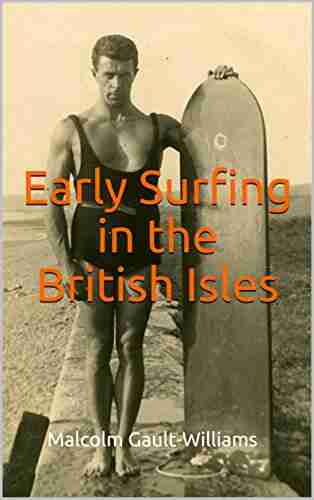

Exploring the Legendary Surfers of Early Surfing in the...
Surfing, a sport...
Light bulbAdvertise smarter! Our strategic ad space ensures maximum exposure. Reserve your spot today!
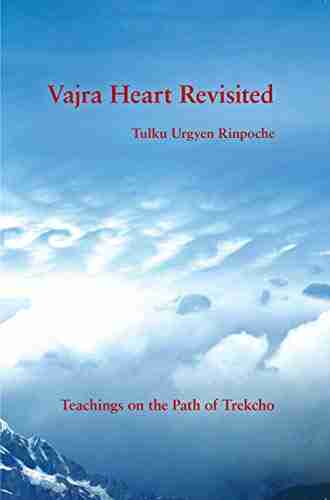

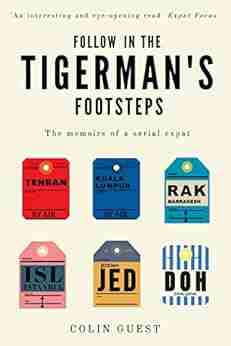

- Aleksandr PushkinFollow ·19.8k
- Seth HayesFollow ·4.6k
- Eli BlairFollow ·2.1k
- Melvin BlairFollow ·18.1k
- Dylan MitchellFollow ·10.1k
- Edward BellFollow ·16.9k
- Edmund HayesFollow ·9.2k
- Preston SimmonsFollow ·12.4k